Your cart is currently empty!
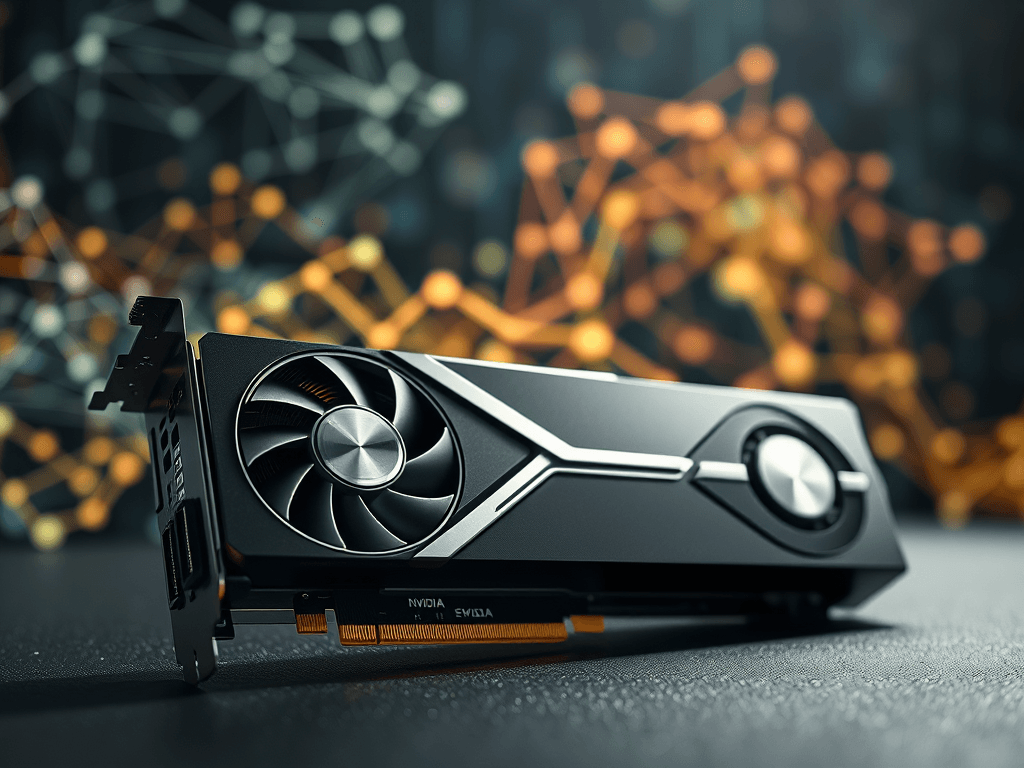
Why NVIDIA Graphics Cards Are Becoming Core for Developing AI Models: A Deep Dive
•
In the rapidly evolving world of artificial intelligence, NVIDIA has emerged as a name that resonates with both researchers and tech enthusiasts. Their GPUs have become an essential component in driving innovation across industries. But what makes NVIDIA graphics cards so pivotal to AI development? Let’s explore the reasons behind their dominance and how they’ve shaped the future of computing.
The Power of Parallelism: Why GPUs Dominate AI
At the heart of AI lies a need for immense computational power. Training neural networks—the core of AI—requires the handling of vast datasets and executing millions of operations per second. Unlike CPUs, which are optimized for sequential tasks, GPUs excel at parallel processing. This distinction is what makes GPUs so effective for AI.
- Matrix Multiplications Made Easy: Neural networks rely heavily on matrix operations. GPUs can perform these operations with unparalleled efficiency.
- High-Throughput Computing: With thousands of cores working in tandem, GPUs process data at lightning speed, slashing training times from weeks to days.
- Seamless Scalability: Whether you’re working with a single GPU or deploying a cluster, NVIDIA’s architecture is built to handle increasingly complex workloads.
What Sets NVIDIA Apart from the Rest?
The GPU market is competitive, but NVIDIA has consistently led the pack. Here’s what makes them the go-to choice for AI developers:
- CUDA: The Developer’s Secret Weapon: CUDA, NVIDIA’s proprietary parallel computing platform, has become a cornerstone for AI development. It simplifies the process of leveraging GPU power, making it accessible for researchers and developers.
- Tensor Cores: NVIDIA introduced Tensor Cores to supercharge AI workloads. These specialized cores accelerate mixed-precision calculations, crucial for training and running neural networks efficiently.
- Robust Software Ecosystem: NVIDIA’s software stack—including cuDNN, TensorRT, and RAPIDS—is designed to integrate seamlessly with popular AI frameworks like TensorFlow and PyTorch.
- Energy Efficiency: AI workloads are power-hungry, but NVIDIA’s GPUs manage to deliver top-tier performance without exorbitant energy costs.
The Real-World Impact of NVIDIA GPUs
The influence of NVIDIA’s GPUs extends across diverse applications. Here are a few examples of how they’re changing the game:
- Training Large Language Models (LLMs): From GPT to BERT, NVIDIA GPUs power the training of some of the most advanced language models in the world. These GPUs handle the massive computations required to process and understand human language.
- Autonomous Driving: NVIDIA’s DRIVE platform is at the forefront of self-driving technology, enabling cars to process real-time data for perception, decision-making, and navigation.
- Healthcare Revolution: In medicine, NVIDIA GPUs are accelerating drug discovery, genomics research, and medical imaging analysis, helping to unlock new treatments and diagnostics.
- Generative AI: NVIDIA’s hardware powers tools that generate realistic images, videos, and even human-like conversations, opening up new possibilities for creativity and automation.
NVIDIA’s A100 and H100: Game-Changers in AI
Among NVIDIA’s product lineup, the A100 and H100 GPUs stand out as groundbreaking innovations:
- A100 (Ampere Architecture): This GPU is a powerhouse for AI training and inference. It’s widely used in data centers to handle complex AI workloads with ease.
- H100 (Hopper Architecture): The H100 takes performance to the next level with its Transformer Engine, which significantly accelerates training for large-scale AI models. It’s tailor-made for applications like LLMs and generative AI.
These GPUs are the backbone of cutting-edge research, enabling breakthroughs that were previously unimaginable.
NVIDIA’s Vision: More Than Just Hardware
NVIDIA isn’t just about making GPUs; it’s about shaping the future of AI. Their initiatives go beyond hardware to include software, platforms, and partnerships:
- NVIDIA AI Enterprise: A comprehensive suite of AI tools designed for businesses to develop, deploy, and manage AI solutions.
- Omniverse: A real-time simulation and collaboration platform that brings virtual worlds to life, enabling AI to be trained in synthetic environments.
- Global Partnerships: Collaborations with cloud providers like AWS, Google Cloud, and Microsoft Azure ensure that NVIDIA’s GPUs are accessible to developers worldwide.
Challenges on the Horizon
Despite their dominance, NVIDIA faces a few hurdles:
- Skyrocketing Demand: As AI adoption grows, meeting the demand for GPUs is a significant challenge.
- Competition: Rivals like AMD and Google (with their TPUs) are pushing hard to claim a share of the AI market.
- Cost Factor: NVIDIA’s high-performance GPUs come with a steep price tag, making them less accessible to smaller players.
The Human Side of NVIDIA’s Journey
One of the most compelling aspects of NVIDIA’s rise is its human impact. The technology they develop is enabling life-saving medical research, advancing accessibility for people with disabilities, and creating opportunities for creatives worldwide. It’s not just about chips and cores—it’s about empowering people to solve real-world problems.
Conclusion: A Future Powered by NVIDIA
NVIDIA’s GPUs have become more than just tools for computation; they’re catalysts for innovation. From revolutionizing industries to driving AI breakthroughs, NVIDIA is shaping the future of technology in profound ways. As AI continues to grow and evolve, NVIDIA’s role will only become more critical.
The question isn’t whether NVIDIA will power the next big thing—it’s how far they’ll take us. Are you ready to explore what’s next?
Discover more from ThunDroid
Subscribe to get the latest posts sent to your email.
Leave a Reply